If LLMs are puppets, who's pulling the strings?
The article from the Mozilla Foundation surfaces into the human decisions that shape generative AI. It highlights the ethical and regulatory implications of these decisions, such as data sourcing, model objectives, and the treatment of data workers.
What gets me about all of this is the ‘black box’ nature of it. Ideally, for example, I want it to be super-easy to train an LLM on a defined corpus of data — such as all Thought Shrapnel posts. Asking questions of that dataset would be really useful, as would an emergent taxonomy.
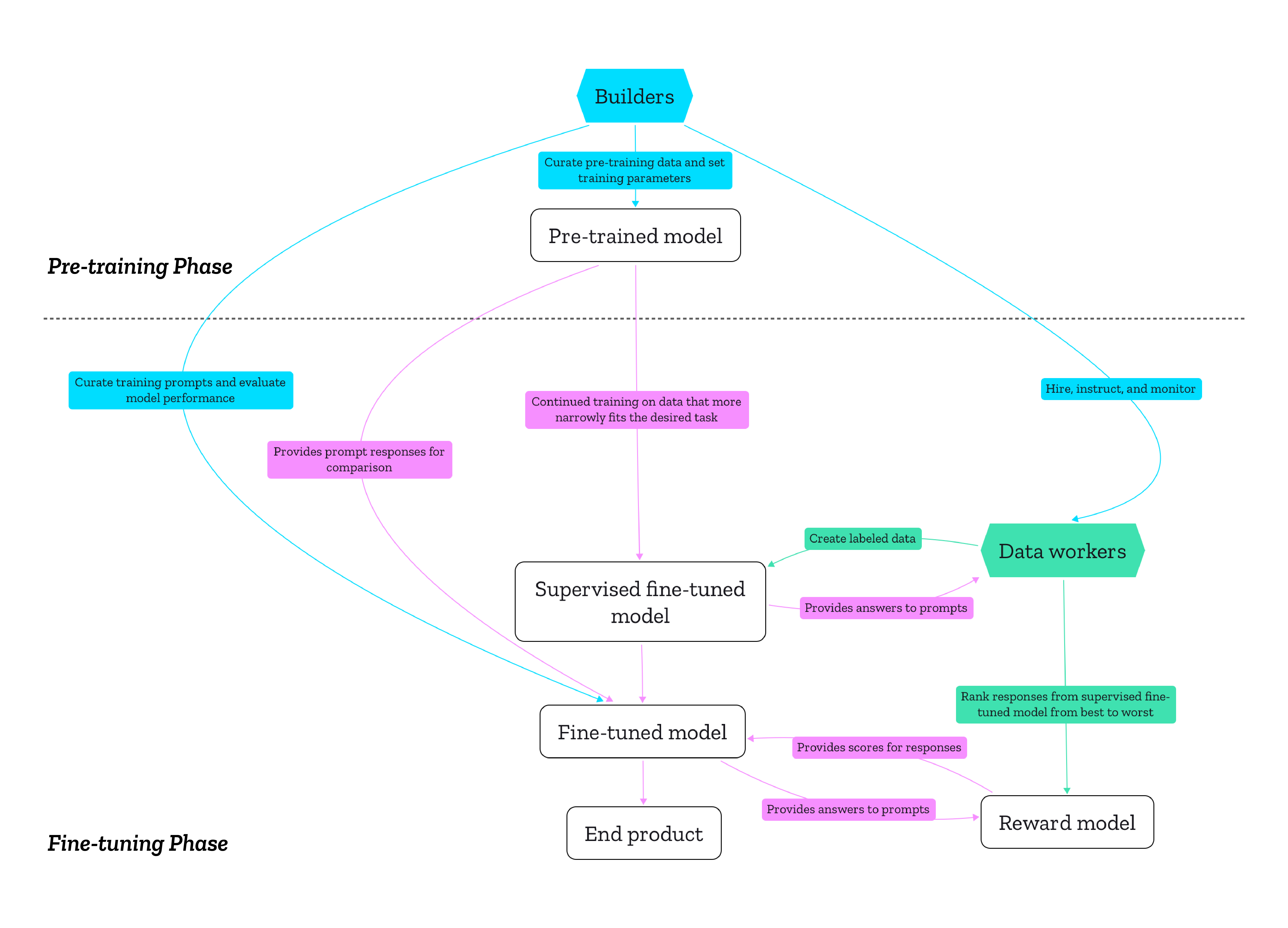
Generative AI products can only be trustworthy if their entire production process is conducted in a trustworthy manner. Considering how pre-trained models are meant to be fine-tuned for various end products, and how many pre-trained models rely on the same data sources, it’s helpful to understand the production of generative AI products in terms of infrastructure. As media studies scholar Luke Munn put it, infrastructures “privilege certain logics and then operationalize them”. They make certain actions and modes of thinking possible ahead of others. The decisions of the creators of pre-training datasets have downstream effects on what LLMs are good or bad at, just as the training of the reward model directly affects the fine-tuned end product.Source: The human decisions that shape generative AI: Who is accountable for what? | Mozilla FoundationTherefore, questions of accountability and regulation need to take both phases seriously and employ different approaches for each phase. To further engage in discussion about these questions, we are conducting a study about the decisions and values that shape the data used for pre-training: Who are the creators of popular pre-training datasets, and what values guide their work? Why and how did they create these datasets? What decisions guided the filtering of that data? We will focus on the experiences and objectives of builders of the technology rather than the technology itself with interviews and an analysis of public statements. Stay tuned!
AI writing detectors don’t work
If you understand how LLMs such as ChatGPT work then it’s pretty obvious that there’s no way “it” can “know” anything. This includes being able to spot LLM-generated text.
This article discusses OpenAI’s recent admission that AI writing detectors are ineffective, often yielding false positives and failing to reliably distinguish between human and AI-generated content. They advise against the use of automated AI detection tools, something that educational institutions will inevitably ignore.
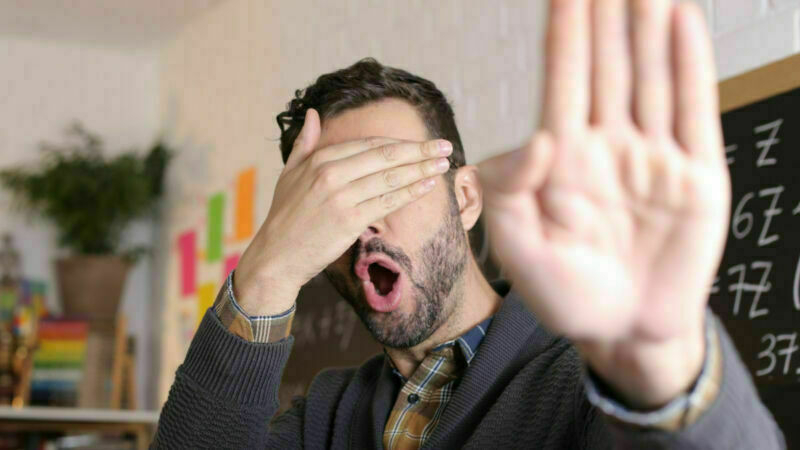
In a section of the FAQ titled "Do AI detectors work?", OpenAI writes, "In short, no. While some (including OpenAI) have released tools that purport to detect AI-generated content, none of these have proven to reliably distinguish between AI-generated and human-generated content."Source: OpenAI confirms that AI writing detectors don’t work | Ars Technica[…]
OpenAI’s new FAQ also addresses another big misconception, which is that ChatGPT itself can know whether text is AI-written or not. OpenAI writes, “Additionally, ChatGPT has no ‘knowledge’ of what content could be AI-generated. It will sometimes make up responses to questions like ‘did you write this [essay]?’ or ‘could this have been written by AI?’ These responses are random and have no basis in fact.”
[…]
As the technology stands today, it’s safest to avoid automated AI detection tools completely. “As of now, AI writing is undetectable and likely to remain so,” frequent AI analyst and Wharton professor Ethan Mollick told Ars in July. “AI detectors have high false positive rates, and they should not be used as a result."
Generative AI, misinformation, and content authenticity
As a philosopher, historian, and educator by training, and a technologist by profession, this initiative really hits my sweet spot. The image below shows how, even before AI and digital technologies, altering the public record through manipulating photographs was possible.
Now, of course, spreading misinformation and disinformation is so much easier, especially on social networks. This series of posts from the Content Authenticity Initiative outlines ways in which the technology they are developing can be prove whether or not an image has been altered.
Of course, unless verification is built into social networks, this is only likely to be useful to journalists and in a court of law. After all, people tend to reshare whatever chimes with their worldview.

Although it varies in form and creation, generative AI content (a.k.a. deepfakes) refers to images, audio, or video that has been automatically synthesized by an AI-based system. Deepfakes are the latest in a long line of techniques used to manipulate reality — from Stalin's darkroom to Photoshop to classic computer-generated renderings. However, their introduction poses new opportunities and risks now that everyone has access to what was historically the purview of a small number of sophisticated organizations.Source: From the darkroom to generative AI | Content Authenticity InitiativeEven in these early days of the AI revolution, we are seeing stunning advances in generative AI. The technology can create a realistic photo from a simple text prompt, clone a person’s voice from a few minutes of an audio recording, and insert a person into a video to make them appear to be doing whatever the creator desires. We are also seeing real harms from this content in the form of non-consensual sexual imagery, small- to large-scale fraud, and disinformation campaigns.
Building on our earlier research in digital media forensics techniques, over the past few years my research group and I have turned our attention to this new breed of digital fakery. All our authentication techniques work in the absence of digital watermarks or signatures. Instead, they model the path of light through the entire image-creation process and quantify physical, geometric, and statistical regularities in images that are disrupted by the creation of a fake.
Can you use CC licenses to restrict how people use copyrighted works in AI training?
TL;DR seems to be that copyright isn’t going to prevent people data mining content to use for training AI models. However, there are protections around privacy that might come into play.
This is among the most common questions that we receive. While the answer depends on the exact circumstances, we want to clear up some misconceptions about how CC licenses function and what they do and do not cover.Source: Understanding CC Licenses and Generative AI | Creative CommonsYou can use CC licenses to grant permission for reuse in any situation that requires permission under copyright. However, the licenses do not supersede existing limitations and exceptions; in other words, as a licensor, you cannot use the licenses to prohibit a use if it is otherwise permitted by limitations and exceptions to copyright.
This is directly relevant to AI, given that the use of copyrighted works to train AI may be protected under existing exceptions and limitations to copyright. For instance, we believe there are strong arguments that, in most cases, using copyrighted works to train generative AI models would be fair use in the United States, and such training can be protected by the text and data mining exception in the EU. However, whether these limitations apply may depend on the particular use case.
We need to talk about AI porn
Thought Shrapnel is a prude-free zone, especially as the porn industry tends to be a technological innovator. It’s important to say, though, that the objectification of women and non-consensual generation of pornography is not just a bad thing but societally corrosive.
By now, we’re familiar with AI models being able to create images of almost anything. I’ve read of wonderful recent advances in the world of architecture, for example. Some of the most popular AI generators have filters to prevent abuse, but of course there are many others.
As this article details, a lot of porn has already been generated. Again, prudishness aside relating to people’s kinks, there are all kind of philosophical, political, legal, and issues at play here. Child pornography is abhorrent; how is our legal system going to deal with AI generated versions? What about the inevitable ‘shaming’ of people via AI generated sex acts?
All of this is a canary in the coalmine for what happens in society at large. And this is why philosophical training is important: it helps you grapple with the implications of technology, the ‘why’ as well as the what. I’ve got a lot more thoughts on this, but I actually think it would be a really good topic to discuss as part of the next season of the WAO podcast.

“Create anything,” Mage.Space’s landing page invites users with a text box underneath. Type in the name of a major celebrity, and Mage will generate their image using Stable Diffusion, an open source, text-to-image machine learning model. Type in the name of the same celebrity plus the word “nude” or a specific sex act, and Mage will generate a blurred image and prompt you to upgrade to a “Basic” account for $4 a month, or a “Pro Plan” for $15 a month. “NSFW content is only available to premium members.” the prompt says.Source: Inside the AI Porn Marketplace Where Everything and Everyone Is for Sale | 404 Media[…]
Since Mage by default saves every image generated on the site, clicking on a username will reveal their entire image generation history, another wall of images that often includes hundreds or thousands of AI-generated sexual images of various celebrities made by just one of Mage’s many users. A user’s image generation history is presented in reverse chronological order, revealing how their experimentation with the technology evolves over time.
Scrolling through a user’s image generation history feels like an unvarnished peek into their id. In one user’s feed, I saw eight images of the cartoon character from the children’s’ show Ben 10, Gwen Tennyson, in a revealing maid’s uniform. Then, nine images of her making the “ahegao” face in front of an erect penis. Then more than a dozen images of her in bed, in pajamas, with very large breasts. Earlier the same day, that user generated dozens of innocuous images of various female celebrities in the style of red carpet or fashion magazine photos. Scrolling down further, I can see the user fixate on specific celebrities and fictional characters, Disney princesses, anime characters, and actresses, each rotated through a series of images posing them in lingerie, schoolgirl uniforms, and hardcore pornography. Each image represents a fraction of a penny in profit to the person who created the custom Stable Diffusion model that generated it.
[…]
Generating pornographic images of real people is against the Mage Discord community’s rules, which the community strictly enforces because it’s also against Discord’s platform-wide community guidelines. A previous Mage Discord was suspended in March for this reason. While 404 Media has seen multiple instances of non-consensual images of real people and methods for creating them, the Discord community self-polices: users flag such content, and it’s removed quickly. As one Mage user chided another after they shared an AI-generated nude image of Jennifer Lawrence: “posting celeb-related content is forbidden by discord and our discord was shut down a few weeks ago because of celeb content, check [the rules.] you can create it on mage, but not share it here.”
CAPTCHA is an arms race we're losing against AI bots
I saw a story that GitHub’s CAPTCHA had become ridiculously hard and multiple people weren’t able to solve it within the time limit. GitHub have presumably upgraded their system because the version we’ve come to know and despise (“click on all of the traffic lights”) is now solved faster by AI than by humans.
“Life is a campaign against malice” said the 17th century Jesuit priest and philosopher Baltasar Gracián. How right he was.

You definitely have tried to access some websites and have gotten bombarded with a series of puzzles requiring you to correctly identify traffic lights, buses, or crosswalks to prove that you’re indeed human before you log in.Source: AI bots are better than humans at solving CAPTCHA puzzles | QuartzKnown as Completely Automated Public Turing test to tell Computers and Humans Apart (CAPTCHA), the technology is intended to protect a website from fraud and abuse without creating friction. The puzzles are meant to ensure that only valid users are able to access the site and not automated invasions.
Google replaced CAPTCHA with a more advanced tool called reCAPTCHA in 2019, but the team’s technical lead Aaron Malenfant told the Verge at the time that the technology would no longer be viable in 10 years’ time because advanced tech would allow the Turing test to run in the background.
His prediction was right. Artificial Intelligence (AI) bots are fast-evolving and are now beating the reCAPTCHA methodology used to confirm the validity and personhood of the users of various websites. They do this by imitating how the human brain and vision work. In fact, AI bots are measuring up to humans, and even beating them, in numerous facets.
Curiosity, projectories, and AI
I’ve read a lot of danah boyd’s work over the years, especially given how her research interests intersect with my work. In this long-ish post, she argues for an approach to AI driven by curiosity and the concept of ‘projectories’ (subject to guardrails).
I just returned from a three month sabbatical spent mostly offline diving through history and I feel like I’ve returned to an alien planet full of serious utopian and dystopian thinking swirling simultaneously. I find myself nodding along because both the best case and worst case scenarios could happen. But also cringing because the passion behind these declarations has no room for nuance. Everything feels extreme and fully of binaries. I am truly astonished by the the deeply entrenched deterministic thinking that feels pervasive in these conversations.Source: Resisting Deterministic Thinking | danah boyd[…]
The key to understanding how technologies shape futures is to grapple holistically with how a disruption rearranges the landscape. One tool is probabilistic thinking. Given the initial context, the human fabric, and the arrangement of people and institutions, a disruption shifts the probabilities of different possible futures in different ways. Some futures become easier to obtain (the greasing of wheels) while some become harder (the addition of friction). This is what makes new technologies fascinating. They help open up and close off different possible futures.
[...]
Even though deterministic thinking can be extraordinarily problematic, it does have value. Studying the scientists and engineers at NASA, Lisa Messeri an Janet Vertesi describe how those who embark on space missions regularly manifest what they call “projectories.” In other words, they project what they’re doing now and what they’re working on into the future in order to create for themselves a deterministic-inflected roadplan. Within scientific communities, Messeri and Vertesi argue that projectories serve a very important function. They help teams come together collaboratively to achieve majestic accomplishments. At the same time, this serves as a cognitive buffer to mitigate against uncertainty and resource instability. Those of us on the outside might reinterpret this as the power of dreaming and hoping mixed with outright naiveté.
[...]
Where things get dicy is where delusional thinking is left unchecked. Guard rails are important. NASA has a lot of guardrails, starting with resource constraints and political pressure. But one of the reasons why the projectories of major AI companies is prompting intense backlash is because there are fewer other types of checks within these systems. (And it’s utterly fascinating to watch those deeply involved in these systems beg for regulation from a seemingly sincere place.)
[...]
Rather than doubling down on deterministic thinking by creating projectories as guiding lights (or demons), I find it far more personally satisfying to see projected futures as something to interrogate. That shouldn’t be surprising since I’m a researcher and there’s nothing more enticing to a social scientist than asking questions about how a particular intervention might rearrange the social order.
[...]
I, for one have no clue what’s coming down the pike. But rather than taking an optimistic or a pessimistic stance, I want to start with curiosity. I’m hoping that others will too.
The madman is the man who has lost everything except his reason
I always enjoy reading L.M. Sacasas' thoughts on the intersection of technology, society, and ethics. This article is no different. In addition to the quotation from G.K. Chesterton which provides the title for this post, Sacasas also quotes Wendell Berry as saying, “It is easy for me to imagine that the next great division of the world will be between people who wish to live as creatures and people who wish to live as machines."
While I’ve chosen to highlight the part riffing off David Noble’s discussion of technology as religion, I’d highly recommend reading the last three paragraphs of Sacasas' article. In it, he talks about AI as being “the culmination of a longstanding trajectory… [towards] the eclipse of the human person”.
The late David Noble’s The Religion of Technology: The Divinity of Man and the Spirit of Invention, first published in 1997, is a book that I turn to often. Noble was adamant about the sense in which readers should understand the phrase “religion of technology.” “Modern technology and modern faith are neither complements nor opposites,” Noble argued, “nor do they represent succeeding stages of human development. They are merged, and always have been, the technological enterprise being, at the same time, an essentially religious endeavor.”Source: Apocalyptic AI | The Convivial Society[…]
The Enlightenment did not, as it turns out, vanquish Religion, driving it far from the pure realms of Science and Technology. In fact, to the degree that the radical Enlightenment’s assault on religious faith was successful, it empowered the religion of technology. To put this another way, the Enlightenment—and, yes, we are painting with broad strokes here—did not do away with the notions of Providence, Heaven, and Grace. Rather, the Enlightenment re-framed these as Progress, Utopia, and Technology respectively. If heaven had been understood as a transcendent goal achieved with the aid of divine grace within the context of the providentially ordered unfolding of human history, it became a Utopian vision, a heaven on earth, achieved by the ministrations Science and Technology within the context of Progress, an inexorable force driving history toward its Utopian consummation.
[…]
In other words, we might frame the religion of technology not so much as a Christian heresy, but rather as (post-)Christian fan-fiction, an elaborate imagining of how the hopes articulated by the Christian faith will materialize as a consequence of human ingenuity in the absence of divine action.
Image: Midjourney (see alt text for prompt)
Bad Bard
Google is obviously a little freaked-out by tools such as ChatGPT and their potentially ability to destroy large sections of their search business. However, it seems like they didn’t do even the most cursory checks of the promotional material they put out as part of the hurried launch for ‘Bard’.
This, of course, is our future: ‘truthy’ systems leading individuals, groups, and civilizations down the wrong path. I’m not optimistic about our future.
In the advertisement, Bard is given the prompt: "What new discoveries from the James Webb Space Telescope (JWST) can I tell my 9-year old about?"Source: Google AI chatbot Bard offers inaccurate information in company ad | ReutersBard responds with a number of answers, including one suggesting the JWST was used to take the very first pictures of a planet outside the Earth’s solar system, or exoplanets. This is inaccurate.
Second-order effects of widespread AI
Sometimes ‘Ask HN’ threads on Hacker News are inane or full of people just wanting to show off their technical knowledge. Occasionally, though, there’s a thread that’s just fascinating, such as this one about what might happen once artificial intelligence is widespread.
Other than the usual ones about deepfakes, porn, and advertising (all which should concern us) I thought this comment by user ‘htlion’ was insightful:
AI will become the first publisher of contents on any platform that exists. Will it be texts, images, videos or any other interactions. No banning mechanisms will really help because any user will be able to copy-paste generated content. On top of that, the content will be generated specifically for you based on "what you like". I expect a backlash effect where people will feel like becoming cattle which is fed AI-generated content to which you can't relate. It will be even worse in the professional life where any admin related interaction will be handled by an AI, unless you are a VIP member for this particular situation. This will strengthen the split between non-VIP and VIP customers. As a consequence, I expect people to come back to localilty, be it associations, sports clubs or neighborhood related, because that will be the only place where they will be able to experience humanity.Source: What will be the second order effects widespread AI? | Hacker News
Generating a logo using an AI drawing model
A couple of weeks ago, I was experimenting with Midjourney and speculating about machine creativity. This post is interesting if you haven’t tried using an AI drawing model as it talks about what Dan Hon calls ‘prompt engineering’ (a term he doesn’t like). Dan also linked to this fantastic example from Andy Baio.
Everybody has heard about the latest cool thing™, which is DALL·E 2 (henceforth called Dall-e). A few months ago, when the first previews started, it was basically everywhere. Now, a few weeks ago, the floodgates have been opened and lots of people on the waitlist got access - that group included me.Source: How I Used DALL·E 2 to Generate The Logo for OctoSQL | Jacob MartinI’ve spent a day playing around with it, learned some basics (like the fact that adding “artstation” to the end of your phrase automatically makes the output much better…), and generated a bunch of (even a few nice-looking) images. In other words, I was already a bit warmed up.
To add some more background, OctoSQL - an open source project I’m developing - is a CLI query tool that let’s you query multiple databases and file formats in a single SQL query. I knew for a while already that its logo should be updated, and with Dall-e arriving, I could combine the fun with the practical.
(Machine) Creativity
It is genuinely amazing what you can create these days with an AI model by simply inputting a few words of natural language. Craiyon (formerly DALL·E mini) allows anyone to do this right now, but there’s also previews of much more powerful models that will be available soon.
As Albert Wenger asks, what does this mean for creative people? I don’t think technology ever completely replaces but rather augments. So I think we’ll see even more artists work with AI models to create amazing things.
During my run today, I was thinking about how awesome it would be to generate running music perfectly suited to the route I was going to run. That’s entirely possible if we continue along this trajectory!
Recently we have had several breakthroughs, first starting with large language models that can tell stories, and now with DALL-E2 and midjourney, two models that can generate amazing imagery based on textual input. For example, here is an image “imagined” by midjourney based on the prompt “Sailing across the alps”Source: The Meaning of Machine Creativity | Continuations by Albert WengerIt is mind-bending to sit with this image for a while. A machine created it and did so within a space of minutes, yet it is full of imagination and detail and could easily be on the cover of a book or the walls of a museum.
So what does it mean that we now clearly and demonstrably have creative machines?
AI-synthesized faces are here to fool you
No-one who’s been paying attention should be in the last surprised that AI-synthesized faces are now so good. However, we should probably be a bit concerned that research seems to suggest that they seem to be rated as “more trustworthy” than real human faces.
The recommendations by researchers for “incorporating robust watermarks into the image and video synthesis networks” are kind of ridiculous to enforce in practice, so we need to ensure that we’re ready for the onslaught of deepfakes.
This is likely to have significant consequences by the end of this year at the latest, with everything that’s happening in the world at the moment…
Synthetically generated faces are not just highly photorealistic, they are nearly indistinguishable from real faces and are judged more trustworthy. This hyperphotorealism is consistent with recent findings. These two studies did not contain the same diversity of race and gender as ours, nor did they match the real and synthetic faces as we did to minimize the chance of inadvertent cues. While it is less surprising that White male faces are highly realistic—because these faces dominate the neural network training—we find that the realism of synthetic faces extends across race and gender. Perhaps most interestingly, we find that synthetically generated faces are more trustworthy than real faces. This may be because synthesized faces tend to look more like average faces which themselves are deemed more trustworthy. Regardless of the underlying reason, synthetically generated faces have emerged on the other side of the uncanny valley. This should be considered a success for the fields of computer graphics and vision. At the same time, easy access (https://thispersondoesnotexist.com) to such high-quality fake imagery has led and will continue to lead to various problems, including more convincing online fake profiles and—as synthetic audio and video generation continues to improve—problems of nonconsensual intimate imagery, fraud, and disinformation campaigns, with serious implications for individuals, societies, and democracies.Source: AI-synthesized faces are indistinguishable from real faces and more trustworthy | PNAS
Generative art
We’re going to see a lot more of this in the next few years, along with the predictable hand-wringing about what constitutes ‘art’.
Me? I love it and would happily hang it on my wall — or, more appropriately, show it on my Smart TV.
Fidenza is my most versatile generative algorithm to date. Although it is not overly complex, the core structures of the algorithm are highly flexible, allowing for enough variety to produce continuously surprising results. I consider this to be one of the most interesting ways to evaluate the quality of a generative algorithm, and certainly one that is unique to the medium. Striking the right balance of unpredictability and quality is a difficult challenge for even the best artists in this field. This is why I’m so excited that Fidenza is being showcased on Art Blocks, the only site in existence that perfectly suits generative art and raises the bar for developing these kinds of high-quality generative art algorithms.Source: Fidenza — Tyler Hobbs
AI-generated misinformation is getting more believable, even by experts
I’ve been using thispersondoesnotexist.com for projects recently and, honestly, I wouldn’t be able to tell that most of the faces it generates every time you hit refresh aren’t real people.
For every positive use of this kind of technology, there are of course negatives. Misinformation and disinformation is everywhere. This example shows how even experts in critical fields such as cybersecurity, public safety, and medicine can be fooled, too.
If you use such social media websites as Facebook and Twitter, you may have come across posts flagged with warnings about misinformation. So far, most misinformation—flagged and unflagged—has been aimed at the general public. Imagine the possibility of misinformation—information that is false or misleading—in scientific and technical fields like cybersecurity, public safety, and medicine.There is growing concern about misinformation spreading in these critical fields as a result of common biases and practices in publishing scientific literature, even in peer-reviewed research papers. As a graduate student and as faculty members doing research in cybersecurity, we studied a new avenue of misinformation in the scientific community. We found that it’s possible for artificial intelligence systems to generate false information in critical fields like medicine and defense that is convincing enough to fool experts.Source: False, AI-generated cybersecurity news was able to fool experts | Fast Company
Mediocrity is a hand-rail
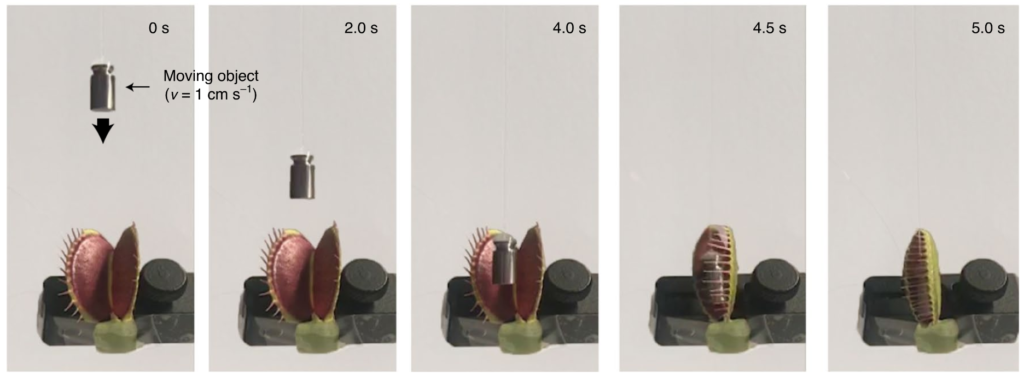
🤖 Engineers Turned Living Venus Flytrap Into Cyborg Robotic Grabber — "The main purpose of this research was to find a way of creating robotic mechanisms able to pick up tiny, delicate objects without harming them. And this particular cyborg creation was able to do just that."
👀 First Look: Meet the New Linux Distro Inspired by the iPad — "This distro is designed to be a tablet first and a “laptop-lite” experience second. And I do mean “lite”; this is not trying to be a desktop Linux distro that runs tablet apps, but a tablet Linux distro that can run desktop ones – a distinction that’s worth keeping in mind."
🤯 DALL·E: Creating Images from Text — "GPT-3 showed that language can be used to instruct a large neural network to perform a variety of text generation tasks. Image GPT showed that the same type of neural network can also be used to generate images with high fidelity. We extend these findings to show that manipulating visual concepts through language is now within reach."
🔊 Surround sound from lightweight roll-to-roll printed loudspeaker paper — "The speaker track, including printed circuitry, weighs just 150 grams and consists of 90 percent conventional paper that can be printed in color on both sides."
👩💻 You can now run Linux on Apple M1 devices — "While Linux, and even Windows, were already usable on Apple Silicon thanks to virtualization, this is the first instance of a non-macOS operating system running natively on the hardware."
Quotation-as-title by Montesquieu. Image from top-linked post.
As scarce as truth is, the supply has always been in excess of demand
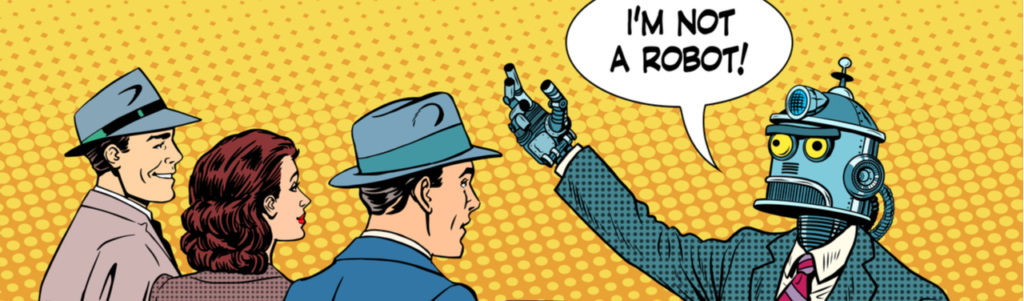
💬 Welcome to the Next Level of Bullshit
📚 The Best Self-Help Books of the 21st Century
💊 A radical prescription to make work fit for the future
👣 This desolate English path has killed more than 100 people
Quotation-as-title by Josh Billings. Image from top linked post.