If LLMs are puppets, who's pulling the strings?
The article from the Mozilla Foundation surfaces into the human decisions that shape generative AI. It highlights the ethical and regulatory implications of these decisions, such as data sourcing, model objectives, and the treatment of data workers.
What gets me about all of this is the ‘black box’ nature of it. Ideally, for example, I want it to be super-easy to train an LLM on a defined corpus of data — such as all Thought Shrapnel posts. Asking questions of that dataset would be really useful, as would an emergent taxonomy.
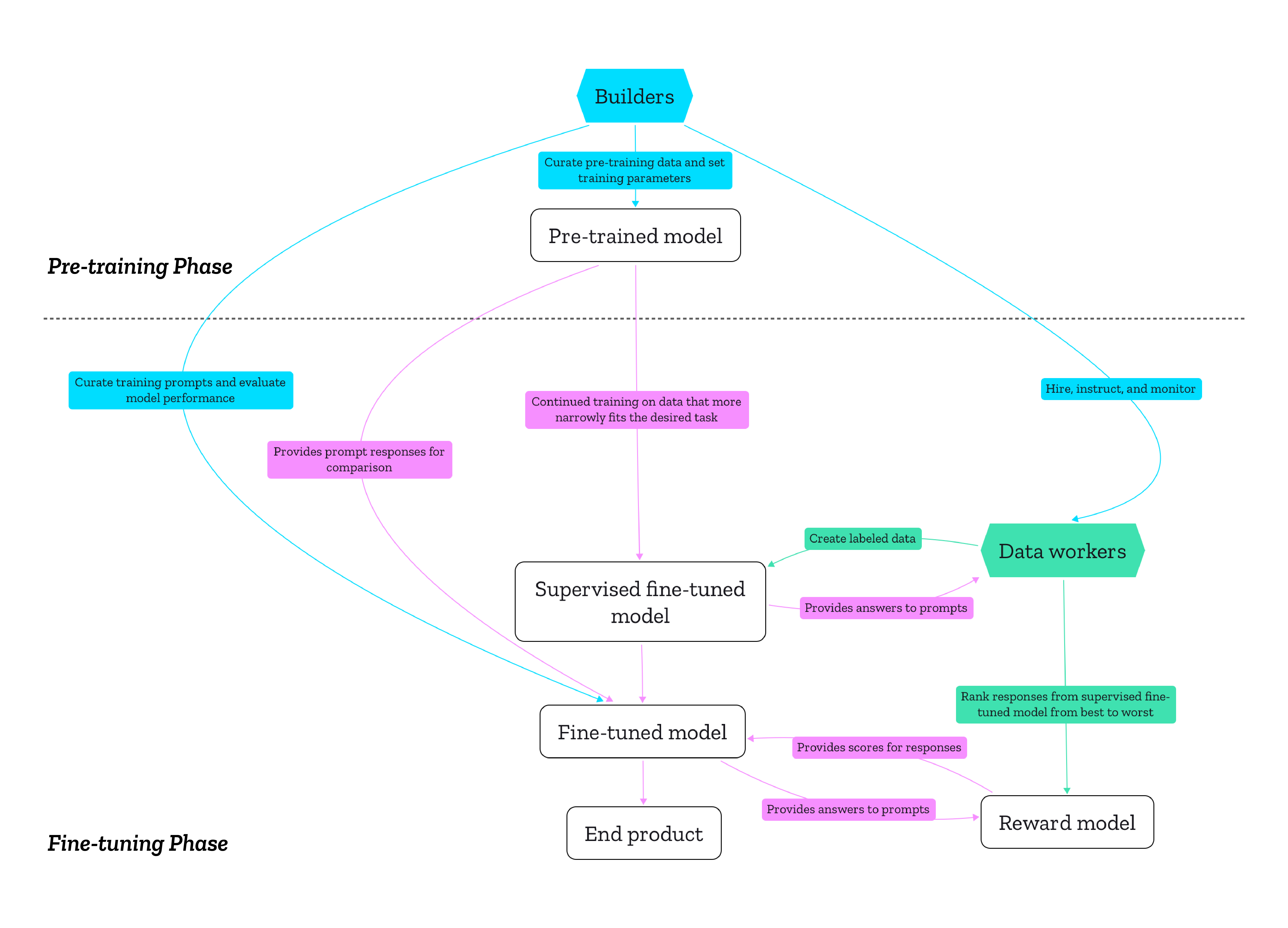
Generative AI products can only be trustworthy if their entire production process is conducted in a trustworthy manner. Considering how pre-trained models are meant to be fine-tuned for various end products, and how many pre-trained models rely on the same data sources, it’s helpful to understand the production of generative AI products in terms of infrastructure. As media studies scholar Luke Munn put it, infrastructures “privilege certain logics and then operationalize them”. They make certain actions and modes of thinking possible ahead of others. The decisions of the creators of pre-training datasets have downstream effects on what LLMs are good or bad at, just as the training of the reward model directly affects the fine-tuned end product.Source: The human decisions that shape generative AI: Who is accountable for what? | Mozilla FoundationTherefore, questions of accountability and regulation need to take both phases seriously and employ different approaches for each phase. To further engage in discussion about these questions, we are conducting a study about the decisions and values that shape the data used for pre-training: Who are the creators of popular pre-training datasets, and what values guide their work? Why and how did they create these datasets? What decisions guided the filtering of that data? We will focus on the experiences and objectives of builders of the technology rather than the technology itself with interviews and an analysis of public statements. Stay tuned!