The occupational classification of a conversation does not necessarily mean the user was a professional in that field
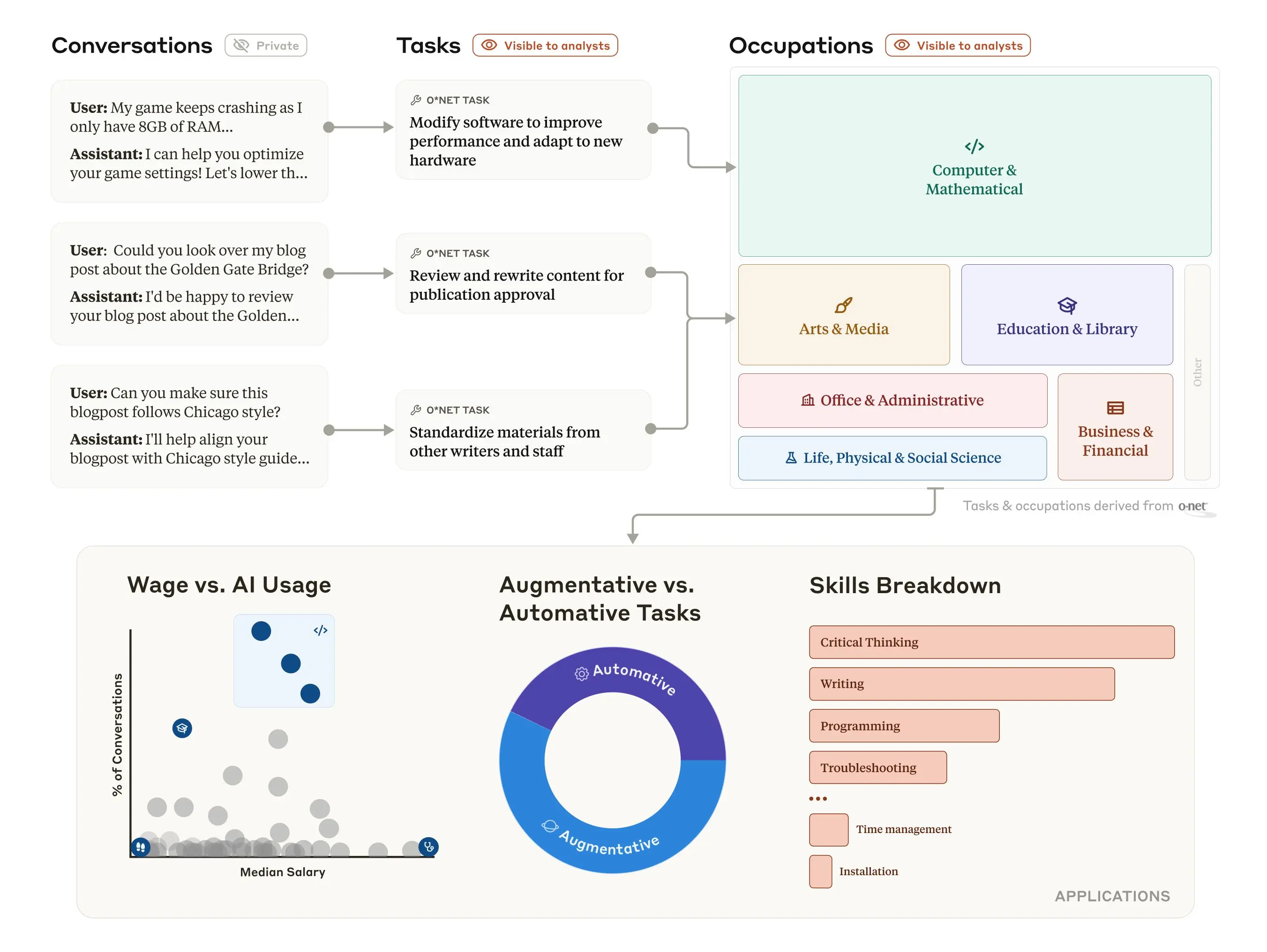
I find this report (PDF) by Anthropic, the AI company behind Claude.ai, really interesting. First, I have to note that they’ve purposely used a report style that looks like it’s been published in an academic journal. But, of course, it hasn’t, which means it’s not peer-reviewed. I’m not saying this invalidates the findings in anyway, especially as they’ve open-sourced the dataset used for the analysis.
Second, although they’ve mapped occupational categories, as the Anthropic researchers point out, “the occupational classification of a conversation does not necessarily mean the user was a professional in that field.” I’ve asked LLMs about health-related things, for example, but I am not a health professional.
Third, and maybe I’m an edge case here, but I use different LLMs for different purposes:
- I primarily use ChatGPT for writing and brainstorming assistance, as well as converting one thing into another. For example, this morning I fed it some PDFs to extract skills frameworks as JSON.
- I use Perplexity when searching for stuff that might take a while to find — for example, the solution a technical problem that might be on an obscure Reddit or Stack Exchange thread.
- I turn to Google’s Gemini if I want to have a conversation with an LLM, say if I’m preparing for a presentation or an interview.
- I use Claude for code-related things because it can create interactive artefacts which can be useful.
- Finally, for sensitive work, or if a client specifically asks, I use Recurse.chat to interact with local LLM models such as LLaVA and Llama.
What I’m saying, I suppose, is that there’s an element of horses for courses with all of this. Increasingly, people will use different kinds of LLMs, sometimes without even realising it. If Anthropic looked at my use of Claude, they’d probably think I had some kind of programming or data analysis job. Which I don’t. So let’s take this with a grain of salt.
The following extract is taken from the report:
Here, we present a novel empirical framework for measuring AI usage across different tasks in the economy, drawing on privacy-preserving analysis of millions of real-world conversations on Claude.ai [Tamkin et al., 2024]. By mapping these conversations to occupational categories in the U.S. Department of Labor’s O*NET Database, we can identify not just current usage patterns, but also early indicators of which parts of the economy may be most affected as these technologies continue to advance.
We use this framework to make five key contributions:
1. Provide the first large-scale empirical measurement of which tasks are seeing AI use across the economy …Our analysis reveals highest use for tasks in software engineering roles (e.g., software engineers, data scientists, bioinformatics technicians), professions requiring substantial writing capabilities (e.g., technical writers, copywriters, archivists), and analytical roles (e.g., data scientists). Conversely, tasks in occupations involving physical manipulation of the environment (e.g., anesthesiologists, construction workers) currently show minimal use.
2. Quantify the depth of AI use within occupations …Only ∼ 4% of occupations exhibit AI usage for at least 75% of their tasks, suggesting the potential for deep task-level use in some roles. More broadly, ∼ 36% of occupations show usage in at least 25% of their tasks, indicating that AI has already begun to diffuse into task portfolios across a substantial portion of the workforce.
3. Measure which occupational skills are most represented in human-AI conversations ….Cognitive skills like Reading Comprehension, Writing, and Critical Thinking show high presence, while physical skills (e.g., Installation, Equipment Maintenance) and managerial skills (e.g., Negotiation) show minimal presence—reflecting clear patterns of human complementarity with current AI capabilities.
4. Analyze how wage and barrier to entry correlates with AI usage …We find that AI use peaks in the upper quartile of wages but drops off at both extremes of the wage spectrum. Most high-usage occupations clustered in the upper quartile correspond predominantly to software industry positions, while both very high-wage occupations (e.g., physicians) and low-wage positions (e.g., restaurant workers) demonstrate relatively low usage. This pattern likely reflects either limitations in current AI capabilities, the inherent physical manipulation requirements of these roles, or both. Similar patterns emerge for barriers to entry, with peak usage in occupations requiring considerable preparation (e.g., bachelor’s degree) rather than minimal or extensive training.
5. Assess whether people use Claude to automate or augment tasks …We find that 57% of interactions show augmentative patterns (e.g., back-and-forth iteration on a task) while 43% demonstrate automation-focused usage (e.g., performing the task directly). While this ratio varies across occupations, most occupations exhibited a mix of automation and augmentation across tasks, suggesting AI serves as both an efficiency tool and collaborative partner.
Source: The Anthropic Economic Index
Image: (taken from the report)