How to Raise Your Artificial Intelligence
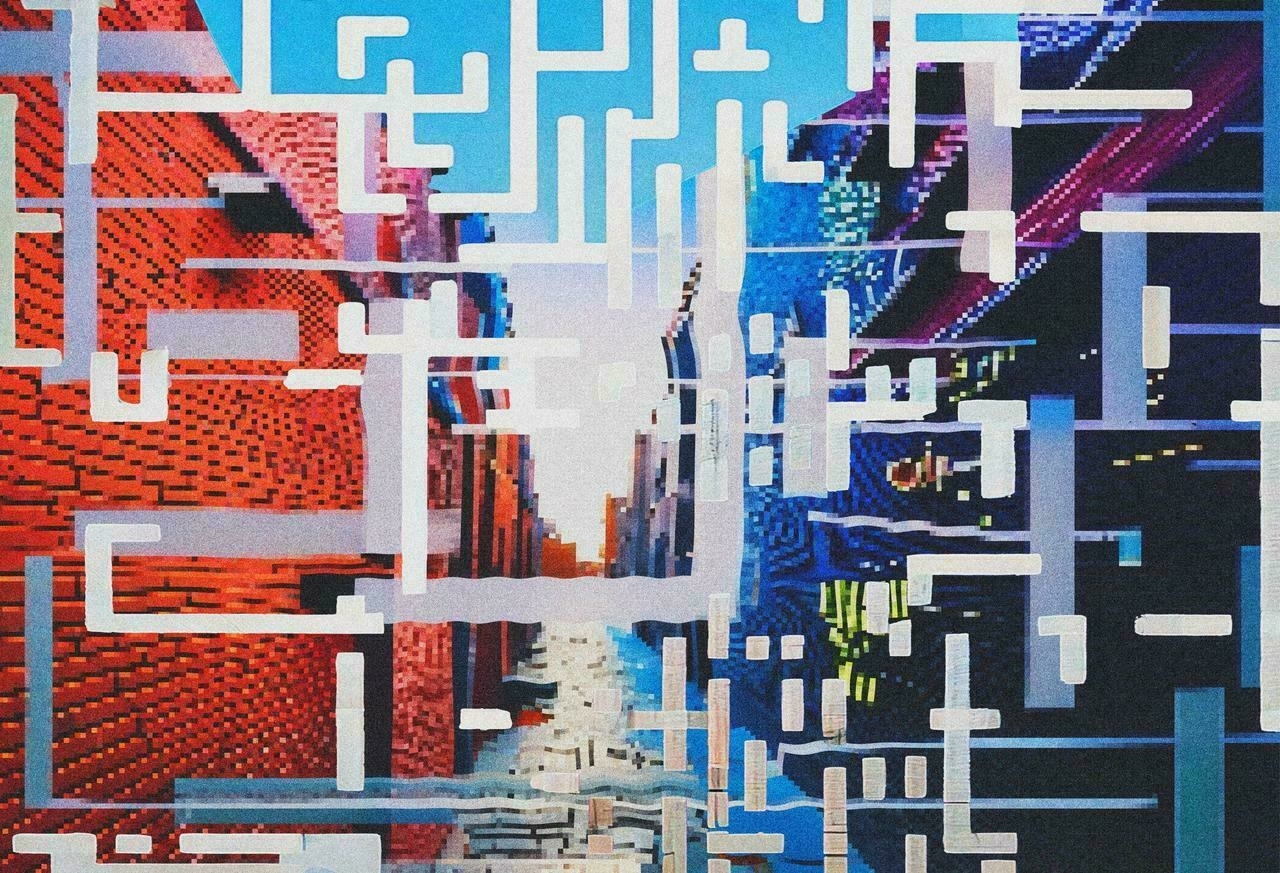
This is an absolutely incredible interview of Alison Gopnik (AG) and Melanie Mitchell (MM). Gopnik is a professor of psychology and philosophy and studies children’s learning and development, while Mitchell is a professor of computer science and complexity focusing on conceptual abstraction and analogy-making in AI systems.
There’s so much insight in here, so you’ll have to forgive me quoting it at length. I urge you to go and read the whole thing. The thing that really stood out for me was Gopnik’s philosophical insights based on her experience around child development. Fascinating.
AG: There is an implicit intuitive model that everyday people (including very smart people in the tech world) have about how intelligence works: there’s this mysterious substance called intelligence, and as you have more of it, you gain power and authority. But that’s just not the picture coming out of cognitive science. Rather, there’s this very wide array of different kinds of cognitive capacities, many of which trade off against each other. So being really good at one thing actually makes you worse at something else. To echo Melanie, one of the really interesting things we’re learning about LLMs is that things like grammar, which we might have thought required an independent-model-building kind of intelligence, you can get from extracting statistical patterns in data. LLMs provide a test case for asking, What can you learn just from transmission, just from extracting information from the people around you? And what requires independent exploration and being in the world?
[…]
MM: I like to tell people that everything an LLM says is actually a hallucination. Some of the hallucinations just happen to be true because of the statistics of language and the way we use language. But a big part of what makes us intelligent is our ability to reflect on our own state. We have a sense for how confident we are about our own knowledge. This has been a big problem for LLMs. They have no calibration for how confident they are about each statement they make other than some sense of how probable that statement is in terms of the statistics of language. Without some extra ability to ground what they’re saying in the world, they can’t really know if something they’re saying is true or false.
[…]
AG: Some things that seem very intuitive and emotional, like love or caring for children, are really important parts of our intelligence. Take the famous alignment problem in computer science: How do you make sure that AI has the same goals we do? Humans have had that problem since we evolved, right? We need to get a new generation of humans to have the right kinds of goals. And we know that other humans are going to be in different environments. The niche in which we evolved was a niche where everything was changing. What do you do when you know that the environment is going to change but you want to have other members of your species that are reasonably well aligned? Caregiving is one of the things that we do to make that happen. Every time we raise a new generation of children, we’re faced with this difficulty of here are these intelligences, they’re new, they’re different, they’re in a different environment, what can we do to make sure that they have the right kinds of goals? Caregiving might actually be a really powerful metaphor for thinking about our relationship with AIs as they develop. […]
Now, it’s not like we’re in the ballpark of raising AIs as if they were humans. But thinking about that possibility gives us a way of understanding what our relationship to artificial systems might be. Often the picture is that they’re either going to be our slaves or our masters, but that doesn’t seem like the right way of thinking about it. We often ask, Are they intelligent in the way we are? There’s this kind of competition between us and the AIs. But a more sensible way of thinking about AIs is as a technological complement. It’s funny because no one is perturbed by the fact that we all have little pocket calculators that can solve problems instantly. We don’t feel threatened by that. What we typically think is, With my calculator, I’m just better at math. […]
But we still have to put a lot of work into developing norms and regulations to deal with AI systems. An example I like to give is, imagine that it was 1880 and someone said, all right, we have this thing, electricity, that we know burns things down, and I think what we should do is put it in everybody’s houses. That would have seemed like a terribly dangerous idea. And it’s true—it is a really dangerous thing. And it only works because we have a very elaborate system of regulation. There’s no question that we’ve had to do that with cultural technologies as well. When print first appeared, it was open season. There was tons of misinformation and libel and problematic things that were printed. We gradually developed ideas like newspapers and editors. I think the same thing is going to be true with AI. At the moment, AI is just generating lots of text and pictures in a pretty random way. And if we’re going to be able to use it effectively, we’re going to have to develop the kinds of norms and regulations that we developed for other technologies. But saying that it’s not the robot that’s going to come and supplant us is not to say we don’t have anything to worry about. […]
Often the metaphor for an intelligent system is one that is trying to get the most power and the most resources. So if we had an intelligent AI, that’s what it would do. But from an evolutionary point of view, that’s not what happens at all. What you see among the more intelligent systems is that they’re more cooperative, they have more social bonds. That’s what comes with having a large brain: they have a longer period of childhood and more people taking care of children. Very often, a better way of thinking about what an intelligent system does is that it tries to maintain homeostasis. It tries to keep things in a stable place where it can survive, rather than trying to get as many resources as it possibly can. Even the little brine shrimp is trying to get enough food to live and avoid predators. It’s not thinking, Can I get all of the krill in the entire ocean? That model of an intelligent system doesn’t fit with what we know about how intelligent systems work.
Source: LA Review of Books